9th DSP Workshop for In-Vehicle Systems and Safety - The Challenge to Society of Moving Intelligence
August 22-23, 2024, Université Libre de Bruxelles (ULB), Brussels, Belgium
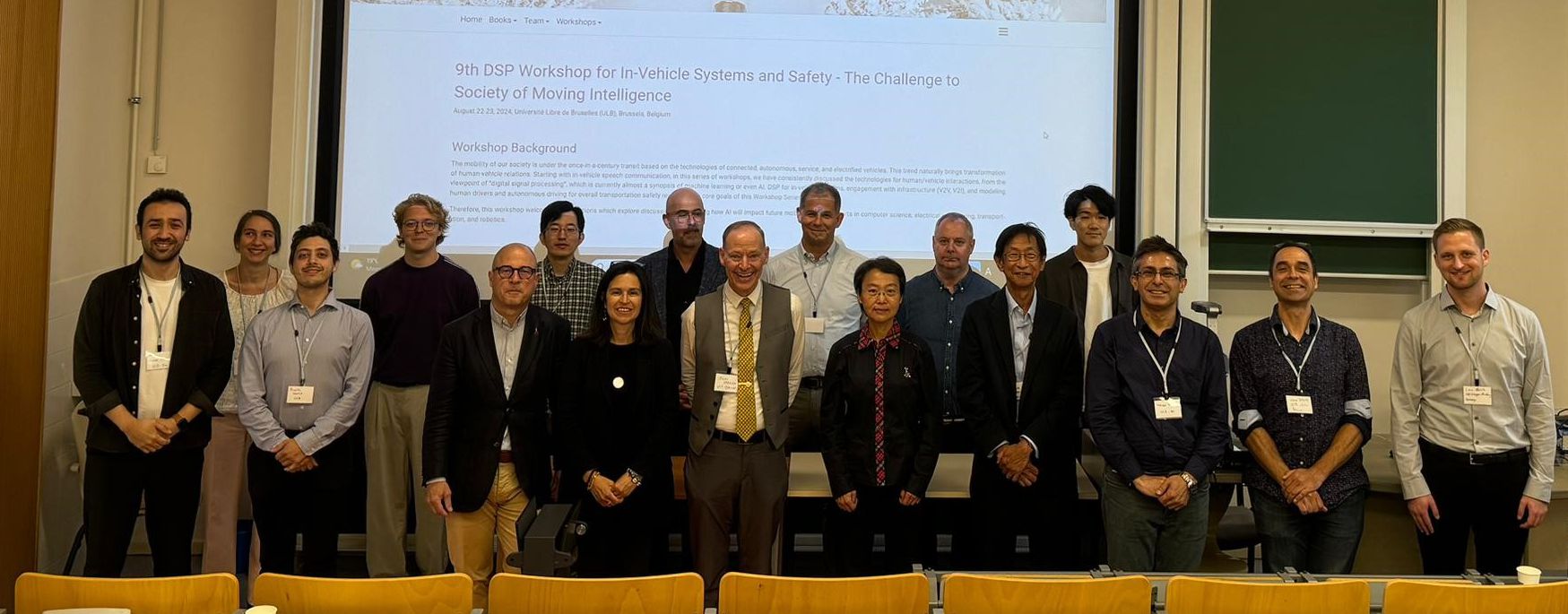
Workshop Background
The mobility of our society is under the once-in-a-century transit based on the technologies of connected, autonomous, service, and electrified vehicles. This trend naturally brings transformation of human-vehicle relations. Starting with in-vehicle speech communication, in this series of workshops, we have consistently discussed the technologies for human/vehicle interactions, from the viewpoint of “digital signal processing”, which is currently almost a synopsis of machine learning or even AI. DSP for in-vehicle systems, engagement with infrastructure (V2V, V2I), and modeling human drivers and autonomous driving for overall transportation safety represent the core goals of this Workshop Series.
Therefore, this workshop welcomes contributions which explore discussions concerning how AI will impact future mobility, among experts in computer science, electrical engineering, transportation, and robotics.
Invitation for Book Chapters
As in the previous workshops, selected presentations in the workshop will be invited for a book chapter in the 4th volume of the Book series, Intelligent Vehicles and Transportation, to be published by Walter de Gruyter publishers.
Workshop History and Format
The organizing committee is pleased to announce the resumption of the Biennial Workshop after more than three years of waiting due to COVID, which take place in Université Libre de Bruxelles (ULB), Brussels, Belgium.
DSP for In-Vehicle systems workshop was established in 2003 in Nagoya Japan, and since then, 7 organized meetings have taken place in Europe (Istanbul, Sesimbra, Kiel), USA (Dallas, Berkeley), and Asia (Nagoya, Seoul).
The workshop combines keynote talks, oral and poster presentations, and panel discussions, which facilitate collaborative research and educational opportunities for researchers, engineers, and students, but also provides international venues for policymakers to actively participate, engage, and share best practices for policy and safety in the discussions. Networking has always been promoted through technical and social events during the meetings.
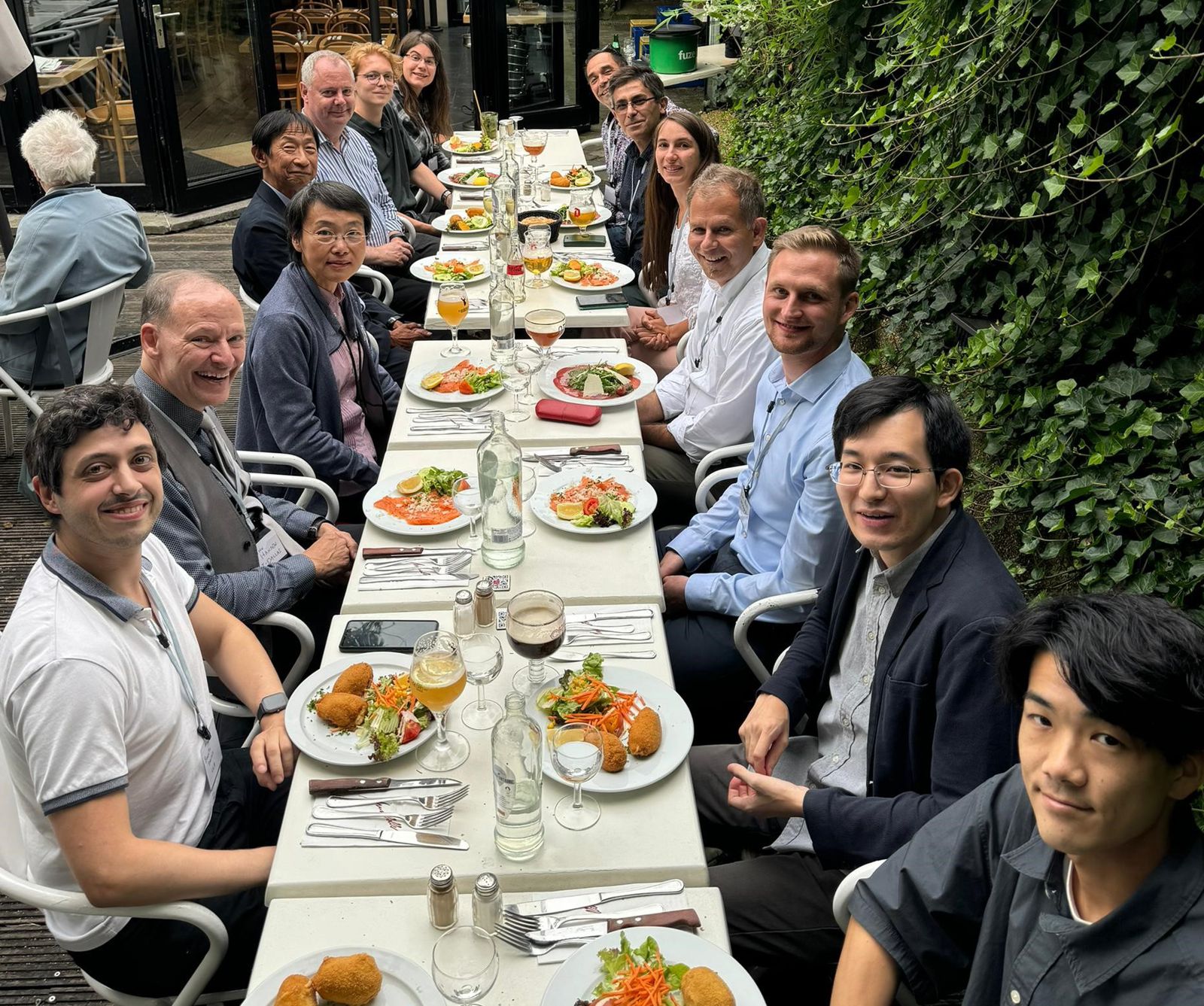
Workshop Topics
- Speech/Acoustics for in-vehicle communications,
- Sensing/Perception for the intelligent vehicles,
- AI/Machine Learning for vehicle intelligence,
- Entertainment/well-being of the mobility,
- 3D image/video processing, communication (Vehicle/transportation),
- 3D visualization and intelligent interface,
- Harmonization/Collaboration among humans, agents, infrastructure,
- New applications and challenges.
Sponsors
- IEEE ITS Society,
- IEEE ITSS Chapter Benelux,
- Université Libre de Bruxelles,
- Nagoya University.
Organizing Committee
- H. Abut (SDSU, USA; Boğaziçi Univ., Istanbul, Türkiye)
- J. Hansen (UTD, Dallas, USA)
- M. Lu (Aeolix ITS, Utrecht, The Netherlands)
- G. Schmidt (CAU, Kiel, Germany)
- K. Takeda (Nagoya U, Nagoya, Japan)
- M. Teratani (ULB, Brussels, Belgium)
Contact
E-mail to
Submission
The intention to contribute should be sent by email to
Workshop Location
Program
Opening (August 22nd, 13:30 h - 14:00 h) |
---|
Session 1 (August 22nd, 14:00 h - 15:40 h) | ||
---|---|---|
Kento Ohtani (1), Tomoki Hayashi (1), Daiki Hayashi (1), Yoshio Ishiguro (2), Kazuya Takeda (3, 4) | (1) Human Dataware Lab, Ltd., (2) The University of Tokyo, (3) Nagoya University, (4) Tier IV inc. |
Driving Scene Understanding and Vehicle ControlUsing Natural Language for Autonomous Driving Services (invited talk)
AbstractAutonomous driving technology has made significant progress, and the widespread use of autonomous driving services is becoming a tangible reality. However, while technological progress is important for the use of autonomous driving services, it is also important the autonomous driving services are widely recognized and accepted by society. In this paper, we discuss two points to improve the social acceptance of autonomous driving services: 1) understanding traffic scenes using natural language in a form that can be easily understood by humans, and 2) controlling autonomous driving vehicles using natural dialogue. Sensor signals acquired from autonomous vehicles are numerical data, and not in a form for people to uniquely understand the situation. Therefore, by describing the driving situation in natural language, it is possible to present what is happening in a way that is easy for people to understand. This makes it possible to appropriately inform the situation, for example, when the automated vehicle comes to an emergency stop. In addition, the use of natural language descriptions facilitates the retrieval of driving data from large driving database. On the other hand, the control of autonomous vehicles is also an important issue to improve the social acceptance. In fully autonomous vehicles, passengers may not control the vehicles at all, and the vehicle may behave in ways that the passengers do not intend. By controlling the vehicle with understanding the passengers’ purpose and intentions through natural dialogue with them, we believe that autonomous vehicles can be used more safely and comfortably. Combined with driving scene understanding, it is also possible to control the vehicle by taking the surrounding environment into account, making the use of autonomous driving services as natural as if a taxi driver were present. |
L. Ullrich (1), A. McMaster (1), K. Graichen (1) | (1) Friedrich-Alexander-Universität Erlangen-Nürnberg | Transfer Learning Study of Motion Transformer-based Trajectory Predictions (invited talk) |
Dongyang Li (1), Ehsan Javanmardi (1), Jin Nakazato (1), Manabu Tsukada (1) | (1) University of Tokyo | End-to-end Vision Transformer-based Learning Approach for Autonomous Vehicle Path Planning
AbstractPerforming an unprotected turn in the intersection is a complex scenario for autonomous vehicles. It not only requires a comprehensive understanding of the surrounding environment but also highly relies on the ego vehicle’s current state to make a safe decision. A conventional way to learn end-to-end autonomous driving is imitation learning, which is learning from expert demonstrations. While most imitation learning methods focus on imitating the expert action, they often fail to imitate a complex policy efficiently when the ego vehicle’s states are crucial to the scenario because there might be arbitrary optimal actions under different states. In this paper, we present a novel cross-attention enhanced imitation learning approach for end-to-end autonomous driving in unprotected turns, which focuses on capturing the relationships between the ego vehicle’s states and the perception of the environment by a cross-attention transformer. We evaluate our model in AWSIM, an open-source autonomous driving simulator across varying traffic densities, and the results demonstrate that our model outperformed conventional imitation learning-based baselines, even when trained only on a limited number of expert demonstrations, showcasing its ability to imitate a complex policy efficiently. |
Hamed Razavi Khosroshahi (1), Jaime Sancho (2), Gun Bang (3), Gauthier Lafruit (1), Eduardo Juarez (2), Mehrdad Teratani (1) | (1) ULB, Belgium, (2) UPM, Spain, (3) ETRI, S. Korea |
Data Augmentation Technique for Neural Radiance Fields (NeRF) and Applications for Autonomous Vehicles
AbstractNeural Radiance Fields (NeRF) demonstrate impressive capabilities in rendering novel views of specific scenes by learning an implicit volumetric representation from posed RGB images without any depth information. One significant challenge in this domain is the need for a large number of images in the training datasets for neural network-based view synthesis frameworks, which is often impractical in real-world scenarios. Our work addresses the challenge of data augmentation for view synthesis applications. NeRF models require comprehensive scene coverage in multiple views to accurately estimate radiance and density at any point. However, insufficient coverage can limit the model’s ability to interpolate or extrapolate unseen parts of a scene effectively. We introduce a novel pipeline to tackle this data augmentation problem using depth data to add novel, non-existent views to the training sets of NeRF framework. Our experimental results show that proposed approach significantly enhances the quality of the rendered images using the NeRF model, with an average increase of 6.4 dB in Peak Signal-to-Noise Ratio (PSNR) and a maximum increase of 11 dB. This work can be extended by integrating LiDAR cameras and their depth maps, to enhance the quality of the view synthesis process, improving the perception and decision-making capabilities of intelligent vehicles. |
Coffee Break (August 22nd, 15:40 h - 16:00 h) |
---|
Session 2 (August 22nd, 16:00 h - 17:40 h) | ||
---|---|---|
Sarah Fachada (1), Daniele Bonatto (1), Gauthier Lafruit (1), Mehrdad Teratani (1) | (1) ULB, Belgium | Plenoptic Camera for Virtual Reality – Insight to its Applications (invited talk)
AbstractFocussed plenoptic cameras are a type of 3D cameras that, by including a micro-lens array in front of their sensor, allow to capture micro-parallax information in one shot and one sensor. This design overcomes the need for cameras with separate depth and color sensors, stereo cameras, or multi-camera systems. However the particuliar design, and resulting images in plenoptic format (a.k.a. lenslet format), calls for new algorithms to calibrate them, extract their 3D information and render comprehensible output images. This presentation provides an overview of the challenges brought and solved by plenoptic cameras, with a focus on virtual reality and telerobotic applications. |
Yafu Tian (1), Alexander Carballo (1, 2, 3) Ruifeng Li (1), Kazuya Takeda (1, 2, 3) | (1) Nagoya University, (2) Gifu University, (3) Tier IV inc. |
RSG-map: An Unified HD-map Representation based on Topological Graph |
Eline Soetens (1), Gauthier Lafruit (1), Mehrdad Teratani (1) | (1) ULB, Belgium | Lenslet Video Compression for Light Field Data and Applications for Autonomous Vehicles
AbstractPlenoptic cameras are able to capture the light field of a scene in a single shot, acquiring plenoptic (aka. lenslet) images and videos which are composed of micro-images. Lenslet videos contain spatial and angular information and can be used to give an immersive experience to remote users. The structure of lenslet images differs from those captured by traditional 2D cameras and is not optimized for traditional video codecs. Existing solutions propose a processing scheme to make the lenslet images more codec-friendly, namely cutting and aligning the micro-images present in the lenslet images. Here we propose an extension to this solution by introducing a smoothing transform in the processing pipeline. The enhanced scheme has been tested on the first 30 frames on 4 sequences and improves the coding performance by 8.97 %. |
Laurie Van Bogaert (1), Gauthier Lafruit (1), Mehrdad Teratani (1) | (1) ULB, Belgium | Depth Image Based Rendering Method to Offer Immersive Experience for Remote Users
AbstractNovel View synthesis methods allow to synthesize novel views from existing capture of a scene. Such methods are useful for autonomous driving development as it allows to create datasets or simulations using existing data captured in the real-world. Moreover, it can be used to place virtually a user in a scene, allowing them to experience safely a critical situation. In particular, Depth Image based rendering (DIBR) can be used to offer an immersive experience to a user using a sparse set of input containing color and depth information. This talk explains the challenges linked of real-time depth image based rendering. It also presents a work in progress of a real-time depth image based rendering software as an example. |
Session 3 (August 23rd, 08:30 h - 10:10 h) | ||
---|---|---|
Daniele Bonatto (1), Sarah Fachada (1), Gauthier Lafruit (1), Mehrdad Teratani (1) | (1) ULB, Belgium | From Point Cloud to 3D Gaussian Splatting – A Review of the State-of-the-art (invited talk) |
Yuto Kumamoto (1), Kento Ohtani (1), Daiki Suzuki (2), Minori Yamataka (12), Kazuya Takeda (1) | (1) Nagoya University (2) DENSO CORPORATION |
Quantifying Accident Risk by Considering Driver Reaction Delays and Driving Environment
AbstractTwo main factors in an accident while driving are the driving environment and the driver. These two factors are closely related: Driver behavior significantly impacts accident risk, and appropriate driver behavior varies depending on the driving environment. This study proposes a method to quantify comprehensive accident risk by integrating both types of risks. As an initial step, we analyze the driver's reaction delay and estimate the reaction delay from driver behavior to quantify the risk of the driver factor. By reflecting this reaction delay in the conventional method for estimating accident risk of the driving environment factor, we aim to integrate the two types of risks. |
Yuze Jiang (1), Ehsan Javanmard (1), Manabu Tsukada (1), Hiroshi Esaki (1) | (1) University of Tokyo | Roadside LiDAR-Based Cooperative Localization with V2I Communication Enhancements
AbstractRecently advancements of vehicle-to-infrastructure (V2I) communication renders cooperative autonomous driving with infrastructure promising and more powerful than standalone autonomous driving. Infrastructure-enabled collective perception is an excellent example of showing the capabilities of road side units (RSUs). Or approach attempts to utilize the perception data from RSU and V2I communication to help vehicle localization. Via V2I communication, the RSU can acquire prior information about connected vehicle which can reduce the positional error of perception. The improved perception is transmitted via V2I communication to the ego-vehicle for cooperative localization. We evaluated our proposed method in AWSIM and Autoware which shows our method boosts localization accuracy by up to 80 % compared to self-localization using NDT in difficult conditions and remains stable despite network challenges. |
Armand Losfeld (1), Sarah Fachada (1), Daniele Bonatto (1), Toshiaki Fujii (2), Gauthier Lafruit (1), Mehrdad Teratani (1) | (1) ULB, Belgium (2) Nagoya University |
3D Multi-view Displays for Autonomous Vehicles: From Acquisition to Displays
AbstractIn an age where immersive media is rapidly becoming an integral part of our digital experiences, the technologies driving this transformation are more fascinating than ever. They hold significant potential for remote applications, such as the monitoring of autonomous vehicles. Despite their potential, only a few types of 3D displays were widely used. The most popular among them is the head-mounted display, which offers high-quality stereoscopic vision but can be cumbersome for various reasons. Glasses-free 3D displays are therefore seen as the next generation. During this presentation, we will first review the different 3D existing glasses-free displays. Then, discuss the input data and computational time of different rendering methods. At the end, some use cases will be presented with a focus on how they can be helpful in autonomous vehicles and autonomous driving. |
Coffee Break (August 23rd, 10:10 h - 10:25 h) |
---|
Session 4 (August 23rd, 10:25 h - 11:40 h) | ||
---|---|---|
Meng Lu (1) | (1) Principal, Aeolix ITS Utrecht, The Netherlands | In-vehicle Systems Deployment from the Perspective of Standardisation (invited talk) |
Naren Bao (1), Alexander Carballo (2), Manabu Tsukada (1), Kazuya Takeda (3) | (1) University of Tokyo (2) Gifu University (3) Nagoya University |
Data-Driven Risk-Sensitive Control for Modeling Personalized Driving Behavior |
Bin Guo (1), John H.L. Hansen (1) | (1) University of Texas at Dallas | Near-future Driving Behavior Prediction based on Stacked LSTM Networks
AbstractPredicting near-future aggressive driving behavior before it happens can significantly improve traffic safety. With advances in deep learning, models are expected to have a more powerful ability to predict and classify aggressive driving behaviors, allowing people to take proactive actions to avoid accidents. Inspired by the Long-short Term Memory (LSTM) network, we present a novel stacked LSTM system that can predict the velocity for the upcoming couple of seconds. The proposed model has the following contributions:
|
Lunch Break (August 23rd, 11:40 h - 13:00 h) |
---|
Panel Session (August 23rd, 13:00 h - 14:00 h) |
---|
Closing (August 23rd, 14:00 h - 14:30 h) |
---|